Package: causalCmprsk 2.0.0
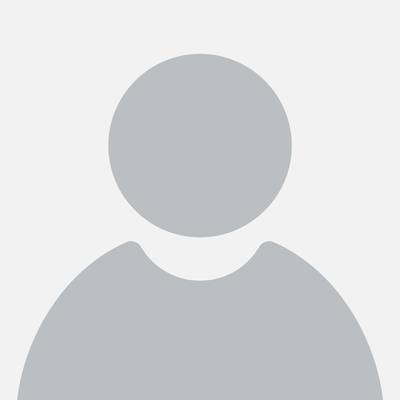
Bella Vakulenko-Lagun
causalCmprsk: Nonparametric and Cox-Based Estimation of Average Treatment Effects in Competing Risks
Estimation of average treatment effects (ATE) of point interventions on time-to-event outcomes with K competing risks (K can be 1). The method uses propensity scores and inverse probability weighting for emulation of baseline randomization, which is described in Charpignon et al. (2022) <doi:10.1038/s41467-022-35157-w>.
Authors:
causalCmprsk_2.0.0.tar.gz
causalCmprsk_2.0.0.zip(r-4.5)causalCmprsk_2.0.0.zip(r-4.4)causalCmprsk_2.0.0.zip(r-4.3)
causalCmprsk_2.0.0.tgz(r-4.4-any)causalCmprsk_2.0.0.tgz(r-4.3-any)
causalCmprsk_2.0.0.tar.gz(r-4.5-noble)causalCmprsk_2.0.0.tar.gz(r-4.4-noble)
causalCmprsk_2.0.0.tgz(r-4.4-emscripten)causalCmprsk_2.0.0.tgz(r-4.3-emscripten)
causalCmprsk.pdf |causalCmprsk.html✨
causalCmprsk/json (API)
# Install 'causalCmprsk' in R: |
install.packages('causalCmprsk', repos = c('https://bella2001.r-universe.dev', 'https://cloud.r-project.org')) |
Bug tracker:https://github.com/bella2001/causalcmprsk/issues
Last updated 1 years agofrom:af47e89026. Checks:OK: 7. Indexed: yes.
Target | Result | Date |
---|---|---|
Doc / Vignettes | OK | Aug 28 2024 |
R-4.5-win | OK | Aug 28 2024 |
R-4.5-linux | OK | Aug 28 2024 |
R-4.4-win | OK | Aug 28 2024 |
R-4.4-mac | OK | Aug 28 2024 |
R-4.3-win | OK | Aug 28 2024 |
R-4.3-mac | OK | Aug 28 2024 |
Exports:fit.coxfit.nonparget.numAtRiskget.pointEstget.weights
Dependencies:clicodetoolsdata.tabledoParallelforeachglueinlineiteratorslatticelifecyclemagrittrMatrixpurrrrlangsurvivalvctrs